Google has just unveiled PaliGemma 2 (Hugging Face and Kaggle), their open-source vision-language technology. Building on the success of the first model, PaliGemma 2 doesn’t just understand words—it can also see and describe images with remarkable clarity. Imagine an AI that not only reads the text but also comprehends and interacts with the visuals surrounding it. This opens up some truly exciting new possibilities for both developers and businesses.
Key Points:
- Enhanced Vision Capability: PaliGemma 2 integrates advanced visual understanding, capturing not just objects but the broader context of images.
- Three Model Sizes and Resolutions: Available in flexible variants, PaliGemma 2 can handle a range of visual tasks, making it versatile for different use cases.
- Simplified Fine-Tuning: With easy integration, PaliGemma 2 allows for customized model fine-tuning that can be tailored to specific needs, right out of the box.
- Broad Use Cases: From medical imaging to creative applications, PaliGemma 2 is demonstrating impressive early results across various fields.
At its core, PaliGemma 2 is a powerful evolution of the earlier Gemma models, extending their functionality to see, understand, and describe the visual world in ways that push the boundaries of AI comprehension. If you remember the original PaliGemma, you’ll recognize the hallmark versatility—only now, it sees in vivid detail.
PaliGemma 2 offers multiple model sizes (3B, 10B, and 28B parameters) and resolutions (224px, 448px, and 896px), giving developers the flexibility to adapt its power to their specific needs. These multiple configurations make PaliGemma 2 suitable for everything from quick, efficient tasks to more complex, demanding visual projects. The model sizes and resolutions are designed to scale, so whether you’re working on something small or a full-scale research project, PaliGemma 2 has you covered.
But what makes PaliGemma 2 genuinely impressive is its ability to generate detailed and contextually rich descriptions of visual scenes. It can recognize actions, emotions, and even the nuanced relationships between objects in an image—essentially adding narrative depth that goes beyond just identifying what’s there. For instance, PaliGemma 2 can generate long, detailed captions for images, making it highly effective for tasks like describing complex medical scans, music scores, or intricate spatial scenes. Google’s technical report highlights its performance on specialized tasks, such as recognizing chemical structures or generating reports from chest X-rays—a testament to its versatility.
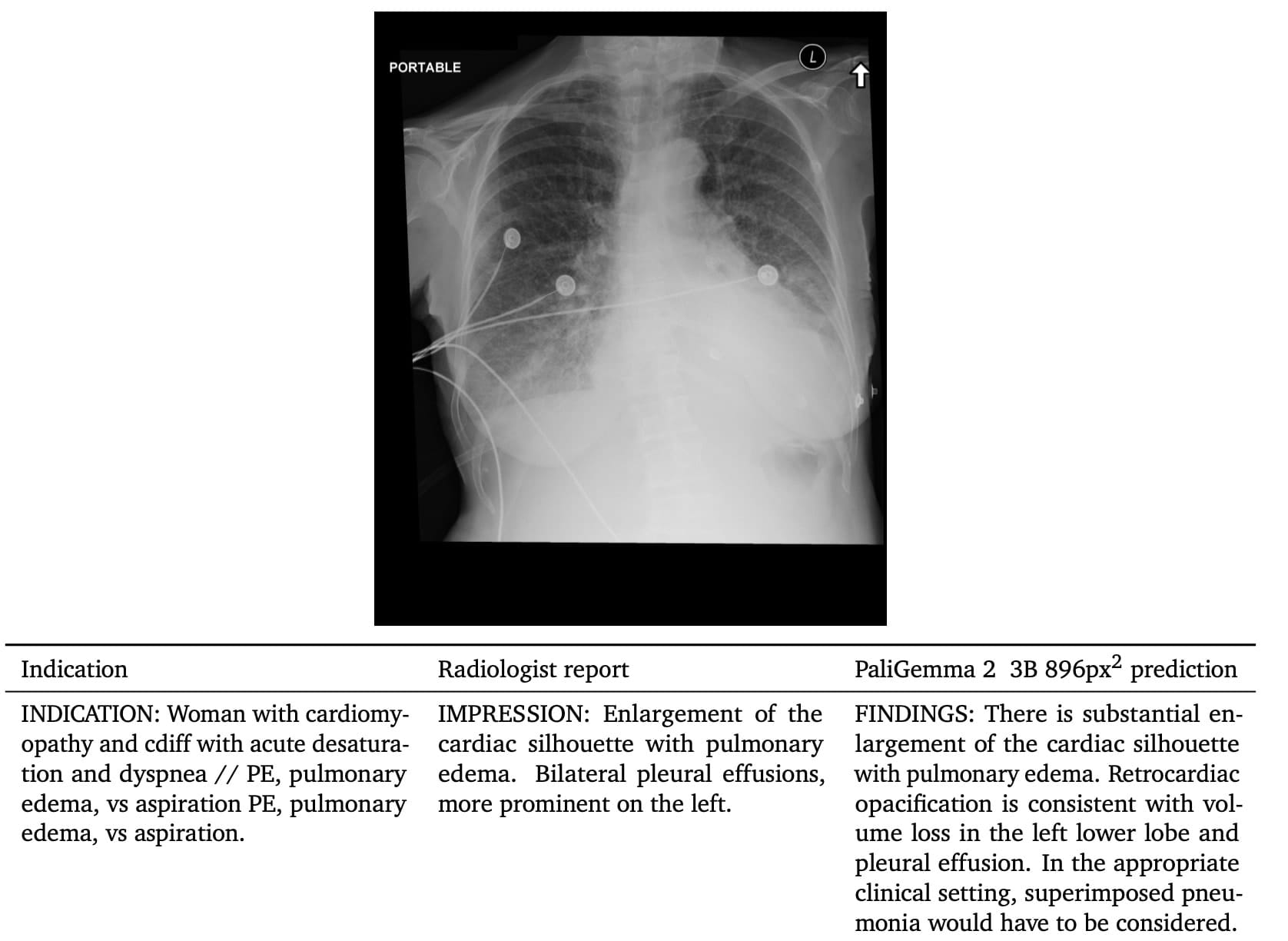
The model builds upon open components like the SigLIP vision model and Gemma 2 language models, and it leverages the architectural recipes of the earlier PaLI-3 model. It uses both an image encoder and a text decoder to understand and then articulate what’s in an image. The result is a system that can answer questions about a picture, identify objects, describe actions, and even provide insights into text embedded within images.
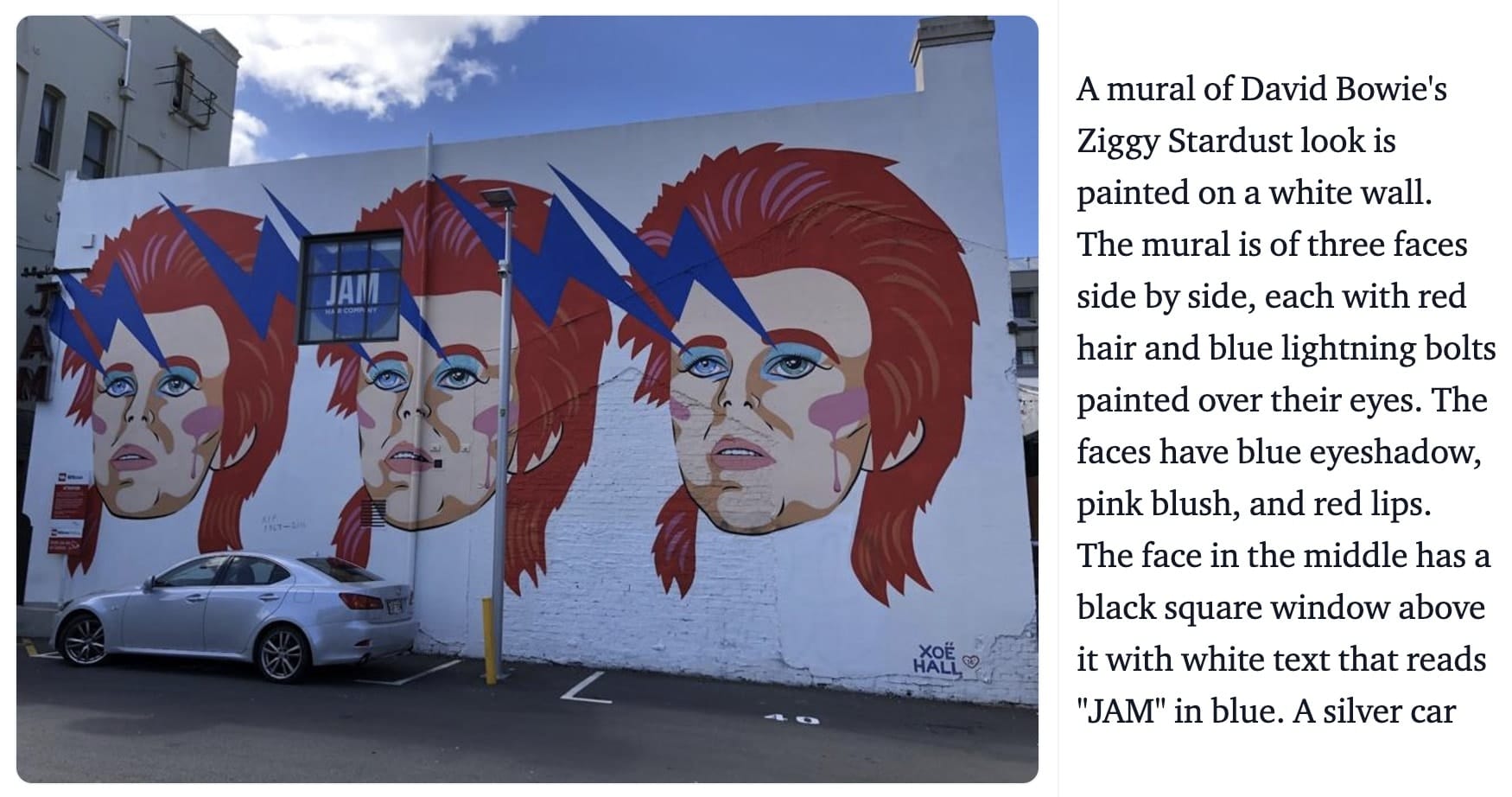
If you used the first PaliGemma model, upgrading to PaliGemma 2 should be a breeze. It’s designed to be a drop-in replacement, providing performance boosts with minimal code changes. Developers who previously fine-tuned PaliGemma can expect to continue using their existing tools and workflows, now with enhanced visual processing and improved model accuracy.
Early experiments using PaliGemma 2 have already shown promising outcomes. For instance, researchers have reported leading results in various benchmark tasks, from general-purpose image captioning to specific applications like document analysis and visual spatial reasoning. Google’s commitment to the Gemmaverse—its ecosystem of models and applications—is evident as PaliGemma 2 expands the frontier of what’s possible with vision-language models.
For those interested in experimenting, PaliGemma 2 is already available for download on platforms like Hugging Face and Kaggle. Developers can use popular frameworks, including PyTorch, Keras, and JAX, to integrate it seamlessly into their projects. Google has also made it easy to get started with sample notebooks and comprehensive documentation that will guide you through setting up inference or fine-tuning tasks.