Google has introduced DataGemma, two new versions of its open-weight Gemma models, that is grounded in real-world statistical data from Google’s Data Commons. The search giant says DataGemma is the first open model that reduces hallucinations by doing this.
Hallucinations are still a big problem with language models. And, this issue is particularly problematic when LLMs are asked to handle numerical or statistical data, where precision is critical.
Google’s Data Commons is a repository of over 240 billion data points collected from trusted organizations like the United Nations and the Centers for Disease Control and Prevention.
By leveraging this massive statistical dataset, DataGemma (which is based on Gemini) is able to significantly improve the accuracy of the model by grounding their outputs in real-world, trustworthy information.
At the heart of DataGemma’s approach are two key techniques: Retrieval-Interleaved Generation (RIG) and Retrieval-Augmented Generation (RAG). Both methods reduce hallucinations by grounding the models in real-world data during the generation process.
RIG operates by proactively querying trusted sources before generating a response. When prompted, DataGemma identifies statistical data points within the query and retrieves accurate information from Data Commons. For instance, if asked, “Has the use of renewables increased globally?”, the model interleaves real-time statistics into its response, ensuring factual accuracy.
RAG, on the other hand, takes this a step further by retrieving relevant information from Data Commons before generating a response. With its long context window (enabled by Gemini 1.5 Pro), DataGemma ensures comprehensive answers, pulling in tables and footnotes that provide deeper context and fewer hallucinations.
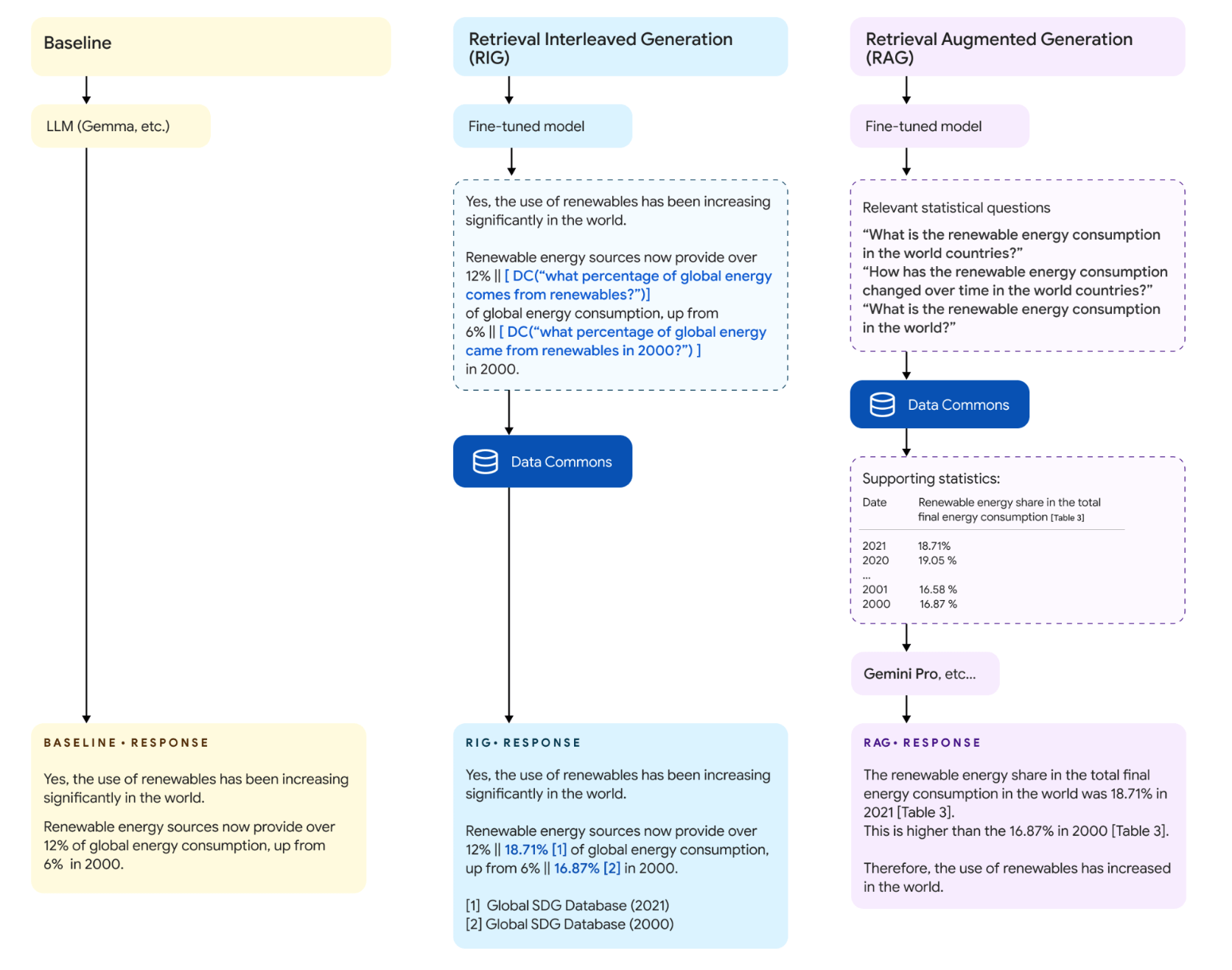
Google’s research into RIG and RAG is still in its early stages, but the initial results are promising. By embedding real-world data into responses, DataGemma models have shown notable improvements in handling numerical facts and statistical queries. The research team has published a paper detailing their methods, highlighting how these techniques help LLMs discern when to rely on external data versus their internal parameters .
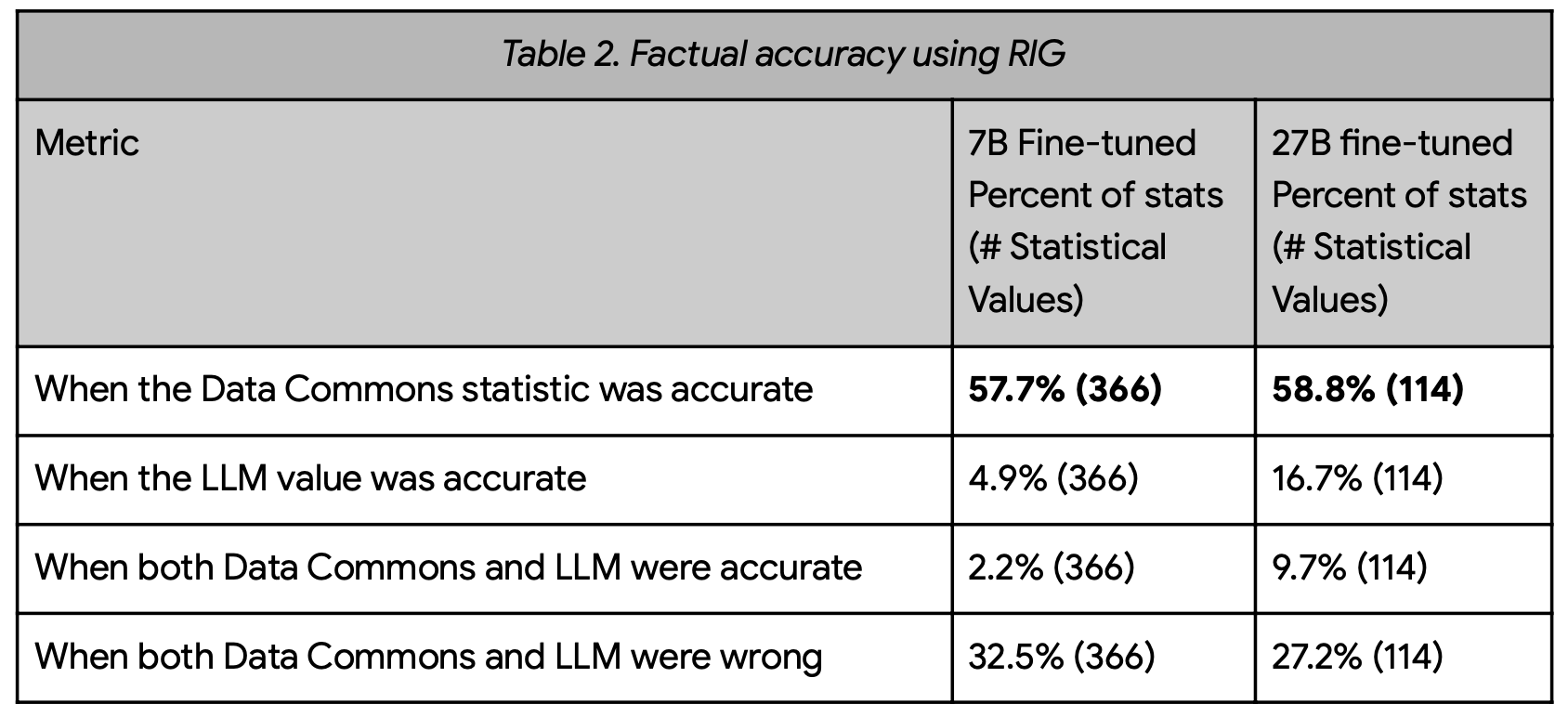
The results of initial testing are promising, albeit with some important caveats. In evaluations using the RIG approach, factual accuracy improved dramatically, jumping from a baseline of 5-17% to about 58%. This represents a significant step forward in the quest for more reliable AI responses. However, the researchers noted that in about 33-27% of cases, both the model and Data Commons provided incorrect information. This was attributed to two main factors: precision issues with the Data Commons natural language interface, and the model generating irrelevant questions.
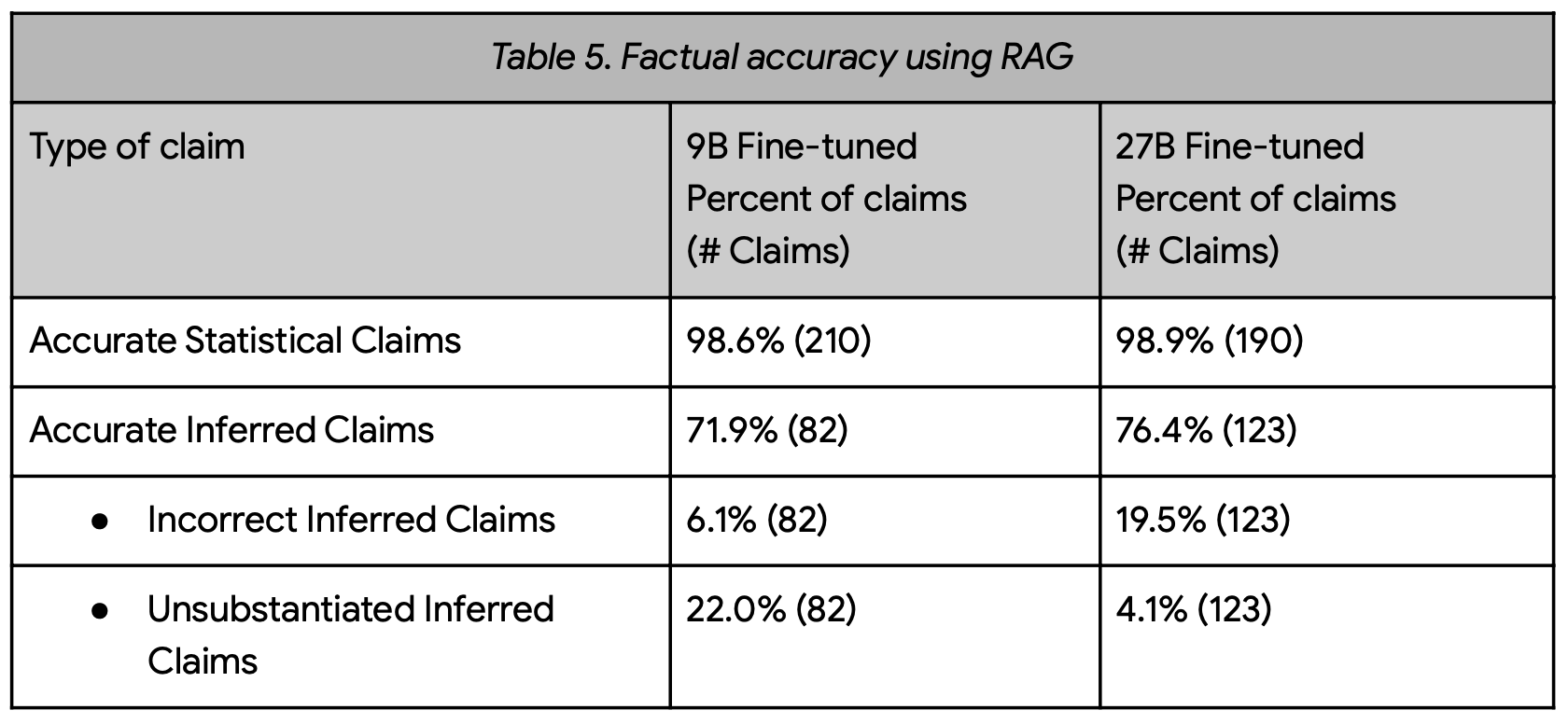
The RAG approach showed even more impressive results in certain areas. When citing specific numerical values from Data Commons, the model achieved an astounding 98-99% accuracy rate. However, the team observed that the model’s performance dropped when it came to drawing inferences based on these statistics, with incorrect or unsubstantiated inferences occurring in 6-20% of cases.
One of the most significant challenges faced by both approaches was data coverage. In many instances, the model was unable to provide a data-grounded response simply because the relevant information wasn’t available in Data Commons. This highlights the ongoing need to expand and refine the datasets available to AI systems.
Despite these challenges, the potential impact of DataGemma is hard to overstate. By providing a mechanism for AI to ground its responses in verifiable, real-world data, Google is taking a significant step towards creating more trustworthy and reliable AI systems. This could have far-reaching implications across a wide range of fields, from healthcare and policy-making to education and scientific research.
However, Google is quick to emphasize that this is an early release, primarily intended for academic and research purposes. The model has been trained on a relatively small corpus of examples and may exhibit unintended behaviors. As such, it’s not yet ready for commercial or general public use.
Looking ahead, the DataGemma team has outlined an ambitious roadmap for future development. Key areas of focus include expanding the model’s training dataset, both in terms of quality and quantity, improving the natural language processing capabilities of Data Commons, and exploring various user interfaces for presenting fact-checked results alongside AI-generated content.
The team is also keenly aware of the ethical implications of their work. They’ve conducted red team exercises to check for potentially dangerous queries and are committed to ongoing evaluation and refinement of the model’s behavior.